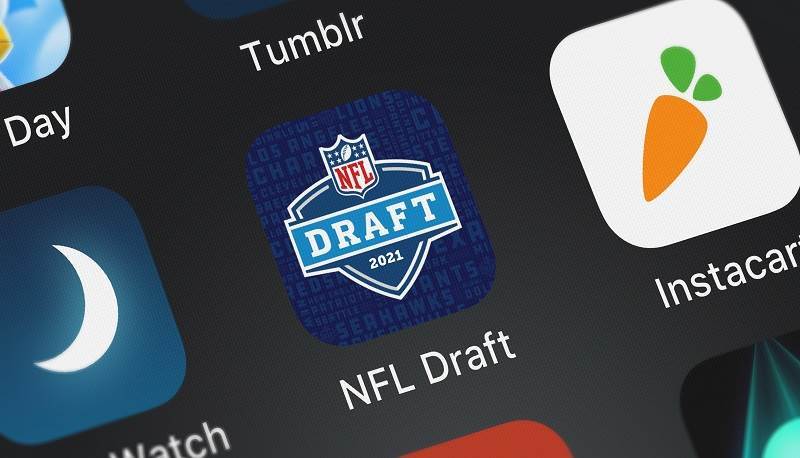
By Steve Cavolick
If it’s April, that means that the NFL Draft is right around the corner. The draft is an integral way teams create their foundation and build a pipeline of talent for future success. That got me thinking about how you build a strong organization that prepares your company for AI.
If I conducted an AI draft, I would create a “Big Board” of capabilities and rank them according to my needs. While my evaluations of AI foundational practices won’t have strengths such as “manhandles defenders in the run game, forklifting or burying once he achieves leverage,” each option would be carefully investigated and rated.
I’ve simulated how I would go about building an organization and Information Architecture that readies my company for a future of AI as a five-round draft of capabilities and skills. Here are my picks:
Round 1 - Prepare your organization for change everywhere
Companies are turning to AI to improve everything from customer service to modeling risk. AI is going to re-write the entire way industries do business. Jobs are going change, and roles are going to become more data-oriented. Humans will need to embrace the model of working alongside automated, intelligent algorithms and look for places where AI can drive improvement. Trying to preserve the “this is how we’ve always done it” approach will leave you in the dust.
Round 2 - A well-rounded team
Teamwork makes the dream work! An AI team does not begin and end with a Data Scientist. SMEs from your company who understand business processes, finance, the competition, and factors effecting your industry are equally important. You want your AI applications to create efficiencies where you need them. You should also have competent Data Operations Specialists and Data Engineers to quickly provide Data Scientists with the quality data they need.
Round 3 - Ethics everywhere
AI models and their predictions are still scary to some people. Make sure you have checks and balances around the use of data and the safeguarding its privacy, that there is transparency in how your models make decisions, and that models are constantly monitored for bias.
Round 4 - Careful project selection
We recommend embarking on many, smaller projects instead of a few massive ones. Select projects where the metric you are trying to influence has a reasonable expectation of change. A single small win often gains momentum and grows into something much more beneficial. With multiple small wins, huge progress can be made with simultaneous, incremental improvement across many areas of the business. The more people/departments that participate and see success, the easier AI adoption will be.
Round 5 - Be prepared for, and learn from, failure
Data scientists can’t predict with 100% certainty how their models will behave once they stop training and are exposed to real-world data. Some models’ confidence scores will erode immediately, while others will degrade over time. Your teams need to set thresholds and continually measure the confidence scores. Even if a model falls one or two percent below the confidence threshold, does that mean it’s time to stop running it and retrain it? Or is it time to scrap the whole thing? These are questions your AI team must decide how to handle.
The LRS Big Data and Analytics group has over 20 years of experience implementing applications in advanced analytics, information management, and data warehousing. Not sure how to get started? Our strategic offerings can help you align business and technology teams, discover the right use case, and determine an ROI. If you are interested in understanding how we can help you find value in your data, please fill out the form below to request a meeting.
About the author
Steve Cavolick is a Senior Solution Architect with LRS IT Solutions. With over 20 years of experience in enterprise business analytics and information management, Steve is 100% focused on helping customers find value in their data to drive better business outcomes. Using technologies from best-of-breed vendors, he has created solutions for the retail, telco, manufacturing, distribution, financial services, gaming, and insurance industries.