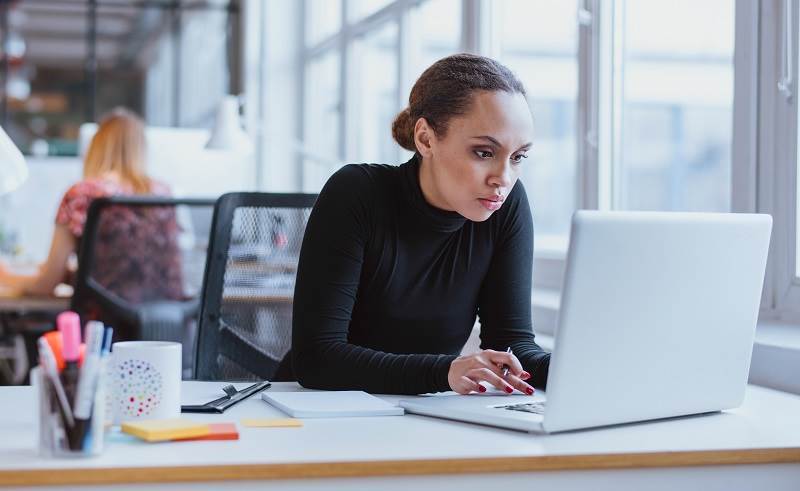
By Steve Cavolick
Innovative organizations realize that AI represents competitive advantage and market differentiation. AI promises to deliver efficiencies and cost savings through faster, more accurate decision making that mimics human thinking. But human thinking can be influenced through individual biases that are often unconscious. One of the largest challenges for data scientists today is discovering and monitoring bias in their predictive models.
There have already been examples where AI applications influenced by bias learned to favor male job applicants over females, and incorrectly determined who is at risk for recidivism.
Bias comes in many forms, including:
- Historical Bias: When biases from the socio-economic and socio-technical conditions of the world enter the data generation process.
- Representation Bias: This happens in the way we define and sample from a population. Data without diversity will exhibit bias.
- Measurement Bias: The way we choose, use, and measure a particular attribute.
- Population Bias: This is when demographic and user characteristics of the data set are different from those in the original target population.
- Social Bias: This kind of bias occurs when other people’s actions affect our own judgement and decisions. Did you ever want to give a restaurant or movie a low rating, but upon observing other highly-rated reviews, think that you may be too critical and change your rating to something less negative?
Because of the potential for bias, the “black box” of AI needs to be governed and explainable. That’s where tools like IBM’s OpenScale can help. OpenScale enables enterprises to enforce fairness in their model’s outcome by analyzing transactions, at both build and runtime, to find biased behavior in the model. It pinpoints the source of bias and actively mitigates the biases found in the production environment. OpenScale works with models built in IBM Watson Machine Learning and most third-party IDEs.
OpenScale has the ability to show us when AI can deployed by itself or when human intervention is still needed. In addition, when biases are discovered in AI, it reveals where biases may exist in our own manual decision making, which may have gone unnoticed for years and years. Bias detection and mitigation in AI models can help show us how human-driven processes can also be tuned in the future.
No matter where you are on your journey to AI, the trustability and traceability of your data and predictive models are foundational characteristics of analytical best practices.
If you have already built AI applications and would like to understand how OpenScale can help you govern them, we can help. If you’re not quite there yet, the LRS Big Data and Analytics team has over 20 years of experience deploying applications in advanced analytics, information management, and data warehousing.
Not sure how to get started? Our strategic offerings can help you align business and technology teams, discover right use case, and determine an ROI. If you are interested in understanding how we can help you find value in your data, please fill out the form below to request a meeting.
About the author
Steve Cavolick is a Senior Solution Architect with LRS IT Solutions. With over 20 years of experience in enterprise business analytics and information management, Steve is 100% focused on helping customers find value in their data to drive better business outcomes. Using technologies from best-of-breed vendors, he has created solutions for the retail, telco, manufacturing, distribution, financial services, gaming, and insurance industries.