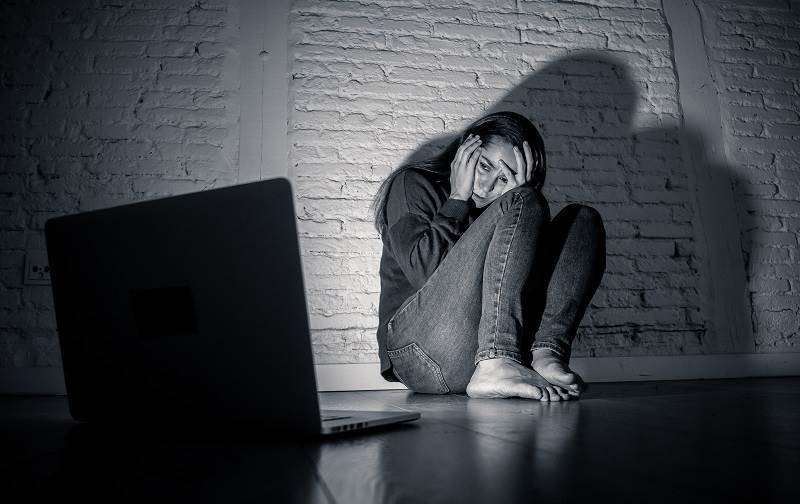
In the history of humankind, few inventions have had the impact on our lives that the internet has. It’s revolutionized the way we conduct business, do banking and travel, consume entertainment, and deliver healthcare. It has also created new avenues for socializing and keeping in touch.
GenZ already relied heavily on social media to communicate, but screen time increased during the pandemic as social distancing required people to reduce physical interactions. Instagram, TikTok, Facebook, WhatsApp, and YouTube are extremely useful, but there is a dark side to them: cyberbullying.
According to stopbullying.gov, cyberbullying includes “sending, posting, or sharing negative, harmful, false, or mean content about someone else. It can include sharing personal or private information about someone else causing embarrassment or humiliation.” And it’s more common than you think.
The National Center For Crime Prevention found that 43% of teenagers had been cyberbullied in the past year, and the effects are greater than just hurt feelings. The biggest impacts of cyberbullying include social anxiety, depression, suicidal thoughts, and self-harm.
With tech companies under scrutiny to take bullying seriously and movements like AI For Good taking root, social media companies have hurried to use machines to detect and deter harmful interactions. Facebook’s DeepText is one such algorithm, but its construction underscores the difficulty in identifying and removing harmful posts while not being overly restrictive.
DeepText has been used by other platforms who want to curb cyberbullying. Following its release in 2010, the founders of Instagram quickly saw the ugly underbelly of their picture-sharing app, and removed mean comments and banned trolls manually. By 2016, there were over one billion users in 20 different languages, which made the old ways of discovering bullying impossible for humans to do. They used DeepText to find insulting or threatening text in posts.
Training an AI model like that used in DeepText is not easy. For example, it is relatively simple to train a machine to detect pictures with nudity. A person either has clothes on or they don’t. It is much more difficult to train a model on bullying because there are no set definitions of what it comprises. Profanity is easy to detect, but slang is always changing. Cyberbullying rarely occurs with single words, so entire sentences must be analyzed and comprehended.
In addition, intent must also be part of the learning. If someone comments “Awesome job” on a photo you post, that can be a compliment, constructive criticism, or an insult. To overcome difficulties in judging intent, data scientists are analyzing more than just text. They now they track the relationship between parties (Has one ever blocked the other? Do they tag each other often?), whether a person’s handle or user information is similar to one that has already been kicked off the platform, and whether a post is part of a coordinated effort to embarrass or hurt someone.
DeepText and other similar applications are still in their infancy and they are off to a good start, but require access to many kinds of governed data. Social media apps should feel a responsibility to use AI for more than just offering personalized ads; they should use it to make their environments cleaner and safe from harmful content.
If you are interested in learning more about how LRS can help you use all of your data to build AI applications, please contact us to request a meeting. Not there yet? We also offer strategic roadmapping services and can help you build an information architecture that will support your current and future analytical applications.
About the author
Steve Cavolick is a Senior Solution Architect with LRS IT Solutions. With over 20 years of experience in enterprise business analytics and information management, Steve is 100% focused on helping customers find value in their data to drive better business outcomes. Using technologies from best-of-breed vendors, he has created solutions for the retail, telco, manufacturing, distribution, financial services, gaming, and insurance industries.